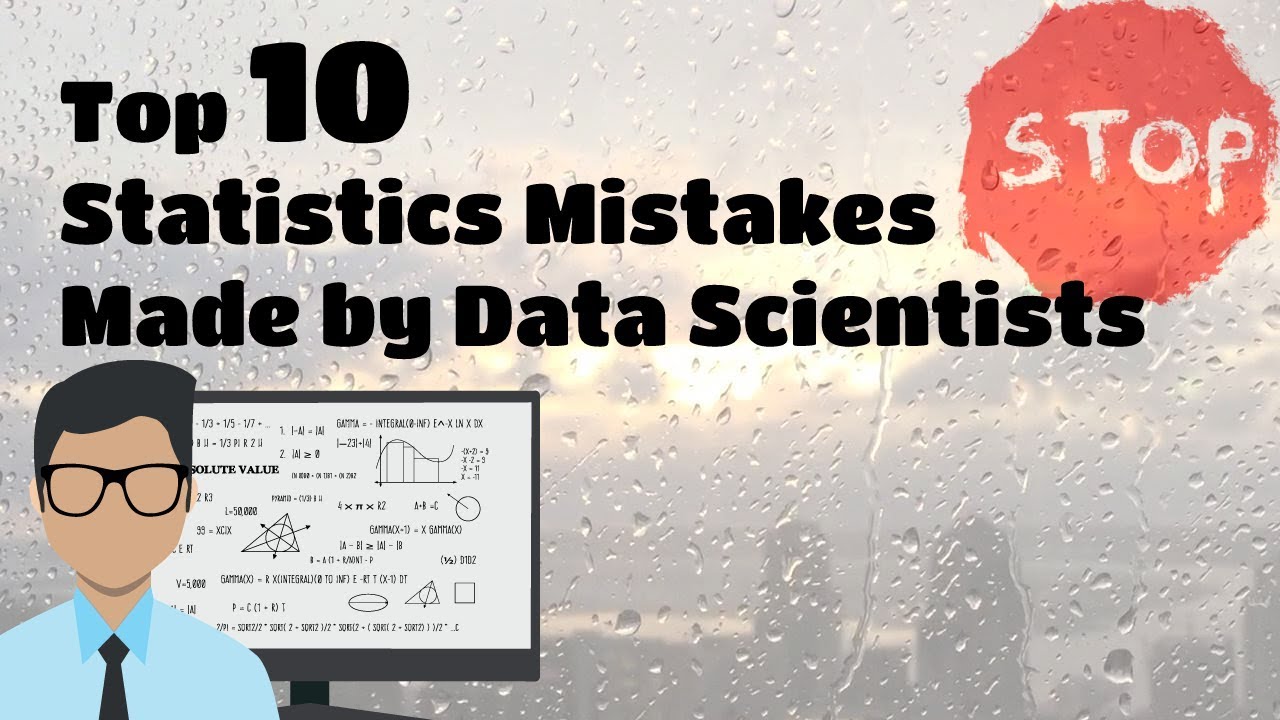
Following mistakes are explained in the video:
#1. Not fully understanding the objective function.
#2. Not having a hypothesis on why something should work.
#3. Not looking at the data before interpreting results.
#4. Not having a naive baseline model.
#5. Incorrect out-sample testing.
#6. Incorrect out-sample testing: applying preprocessing to full dataset.
#7. Incorrect out-sample testing: cross-sectional data & panel data.
#8. Not considering which data is available at point of decision.
#9. Subtle Overtraining.
#10. "need more data" fallacy.
Hoping those data science tips will help you in your carrier and became the best data scientist or data analyst in the company!
Source of material:
0 Comments